Introduction
In the fast-paced world of retail, staying ahead of trends and making data-driven decisions is more critical than ever. With the advent of retail predictive analytics, businesses now have the tools to forecast trends, optimize operations, and improve their overall customer experience. Retail predictive analytics is a key player in the transformation of retail operations, moving away from traditional approaches to a more informed, proactive strategy.
This article will delve into the transformative role of retail predictive analytics, highlighting how it helps businesses make better decisions, improve their store analysis reports, and predict future trends with greater accuracy. The importance of leveraging data analytics for retail cannot be overstated, as it provides actionable insights that drive decisions in everything from inventory management to customer service and marketing strategies.
By using retail store analysis reports, retailers gain clarity on business performance, and through in store analytics, they can enhance customer experiences. Throughout this piece, we will explore the full scope of how retail predictive analytics can provide a competitive edge for businesses, enabling them to stay ahead of the curve.
Quick Access Guide
What Is Retail Predictive Analytics?
Retail predictive analytics is the practice of using historical data, statistical algorithms, and machine learning techniques to predict future outcomes in the retail environment. At its core, it combines the power of advanced analytics with real-time data to provide actionable insights, forecasting everything from customer behavior to inventory needs.
Definition and Key Concepts of Retail Predictive Analytics
Retail predictive analytics allows businesses to anticipate future trends and behaviors based on past data. It uses various types of data such as sales trends, customer preferences, and even external factors like weather patterns and local events to predict consumer purchasing behaviors.
One of the key concepts in retail predictive analytics is the use of predictive modeling. Predictive models analyze data from multiple sources to identify patterns, correlations, and trends, which are then used to make forecasts. These forecasts can inform decisions about stock levels, pricing strategies, promotional offers, and even the optimal store layout.
How Predictive Analytics Differs from Traditional Analytics
Traditional analytics tends to focus on past and present data, offering insights into what has happened. However, retail predictive analytics goes a step further by forecasting what is likely to happen in the future. This proactive approach provides retailers with the foresight they need to plan their strategies accordingly.
Traditional methods might rely on static reports that summarize past sales figures or customer behaviors. In contrast, retail predictive analytics uses dynamic models to predict shifts in consumer behavior, allowing businesses to take action before a trend fully develops. This shift from reactive to proactive decision-making is one of the most significant advantages of predictive analytics in the retail sector.
Importance in Making Data-Driven Decisions
Retail predictive analytics is integral to data-driven decision-making, as it provides evidence-based insights that remove much of the guesswork from retail operations. Retailers can make more accurate forecasts for stock management, which can lead to better inventory control and fewer stockouts or overstock situations. This, in turn, leads to cost savings and greater customer satisfaction.
Moreover, by relying on predictive analytics, retailers can make more informed decisions about marketing strategies, pricing models, and promotional activities. Data analytics for retail helps companies understand which strategies are likely to be most successful based on historical data, enabling more targeted and efficient approaches to customer engagement.
The Role of Data Analytics for Retail
In the retail world, data analytics for retail is an invaluable tool that unlocks insights from raw data. By analyzing large volumes of data from various sources, retailers can identify trends, make predictions, and optimize their operations.
How Data Analytics for Retail Enhances Decision-Making
Data analytics for retail plays a critical role in improving decision-making across all aspects of retail operations. By analyzing customer data, sales patterns, and other relevant information, retailers can identify what products are likely to sell well, when to adjust pricing, and how to optimize inventory. Retailers can also use this data to enhance their marketing efforts, tailoring promotions to specific customer segments.
Retail analytics is an example of this data in action, providing insights that drive everything from operational decisions to customer-facing strategies. With this wealth of data, businesses can make better-informed decisions that lead to improved efficiency and profitability.
Key Areas Where Data Analytics Impacts Retail Operations
- Inventory Management: By analyzing sales trends and customer preferences, retailers can optimize their inventory levels, reducing the risk of overstocking or stockouts.
- Customer Behavior: Through in-store analytics and purchase history, businesses can predict customer preferences, enabling them to create personalized shopping experiences.
- Sales Optimization: Retailers can forecast future sales based on historical data, allowing them to adjust marketing and sales strategies accordingly.
- Supply Chain Efficiency: Data-driven insights help streamline supply chain operations, ensuring that products are delivered at the right time and in the right quantities.
Importance of Collecting Quality Data for Predictive Analytics
The success of retail predictive analytics heavily depends on the quality of the data collected. Inaccurate or incomplete data can lead to misleading insights and ineffective decision-making. Therefore, it is crucial for businesses to ensure that they are gathering accurate, high-quality data from reliable sources.
Retail store analysis reports are a vital tool in ensuring that the data used for predictive analytics is accurate. By continuously collecting and refining data, retailers can ensure that their predictive models provide actionable and reliable insights.
How Retail Predictive Analytics Improves Retail Store Analysis Reports
One of the most significant applications of retail predictive analytics is in the creation of detailed and accurate retail store analysis reports. These reports provide business leaders with critical insights into store performance, allowing them to make informed decisions.
Benefits of Using Predictive Analytics in Generating Accurate Retail Store Analysis Reports
Using retail predictive analytics in store analysis allows businesses to move beyond basic reporting. These reports become more than just summaries of past performance; they evolve into actionable insights that predict future performance.
By incorporating predictive analytics into their store analysis reports, retailers can anticipate future trends and identify potential challenges before they arise. This proactive approach helps businesses make informed decisions about everything from inventory levels to staffing requirements.
Tools and Methods Used for Analysis in Retail Stores
Retailers use a variety of tools to integrate retail predictive analytics into their operations. These include:
- Data visualization tools: These tools help display complex data in an easily digestible format, making it simpler for retailers to understand trends and insights.
- Point-of-sale (POS) systems: POS data is an invaluable source of information for understanding sales patterns and customer behavior.
- Customer Relationship Management (CRM) systems: These systems store detailed customer data, which can be used to predict future purchases and tailor marketing efforts.
Real-World Examples of Improved Store Reports Through Predictive Analytics
Retailers such as Walmart and Target have successfully integrated retail predictive analytics into their operations. For example, Walmart uses predictive analytics to optimize its inventory management, ensuring that the right products are always in stock. This has led to more accurate retail store analysis reports, which in turn have improved sales and customer satisfaction.
Predicting Business Trends with Retail Predictive Analytics
One of the key advantages of retail predictive analytics is its ability to forecast business trends. By analyzing historical data and customer behavior, retailers can predict future demand, shifts in customer preferences, and other business trends that can significantly impact their operations.
How Predictive Analytics Forecasts Customer Behavior and Trends
Predictive analytics uses historical data to create models that predict customer behavior. For example, if a particular product category sees a rise in sales during a specific season, retail predictive analytics can forecast future sales for that category during the same season in the upcoming year. This helps businesses plan their inventory, marketing campaigns, and sales strategies in advance.
In-Store Analytics: Leveraging Predictive Data
In-store analytics is a powerful tool that allows retailers to gain a deeper understanding of their operations and customer behavior. By combining retail predictive analytics with real-time data collection, retailers can enhance both customer experiences and operational efficiency.
How In-Store Analytics Improves Customer Experiences and Operational Efficiency
In-store analytics provides real-time insights into customer movements, dwell times, and product interactions within the store. This data, when combined with retail predictive analytics, helps retailers optimize store layouts, product placements, and staffing levels to improve the customer shopping experience. Predictive models can forecast peak shopping times, allowing retailers to adjust staffing levels accordingly, ensuring that customers are never left waiting.
For example, using retail predictive analytics, stores can predict which areas of the store will have high traffic and which products will likely experience higher demand. This allows businesses to strategically place products where they are most likely to be noticed and purchased, thus improving sales and customer satisfaction.
Real-Time Insights from In-Store Analytics Tools
In-store analytics tools like heatmaps, motion sensors, and foot traffic trackers provide real-time data on customer behavior. These tools allow businesses to gather actionable insights that help optimize store operations. When combined with predictive models, these insights can be used to forecast customer behavior, streamline store operations, and even customize marketing efforts in real time.
For instance, a retailer might use retail predictive analytics to determine that customers tend to spend more time in certain areas of the store during specific times of the day. By using these insights, they can position popular products in these high-traffic areas, increasing the likelihood of sales.
The Synergy Between In-Store Analytics and Predictive Models
The synergy between in-store analytics and predictive models is where the true power lies. By merging real-time data with predictive insights, retailers can optimize their operations in ways that were previously impossible. For example, predictive models can forecast customer preferences based on historical data, and in-store analytics can confirm or adjust these forecasts in real time.
Retailers can use this synergy to adapt quickly to changes in customer behavior, optimize their inventory in real time, and deliver personalized shopping experiences that drive higher sales and customer loyalty.
Retail Analytics: How Predictive Insights Drive Strategy
Retail analytics powered by predictive insights are not just about analyzing past trends—they are about shaping future strategies that drive growth. With the right analytics tools in place, retailers can create more informed and data-driven strategies that improve sales, marketing, and operational performance.
How Retail Analytics Influence Strategic Planning
Predictive analytics in retail analytics plays a vital role in shaping strategic planning. By forecasting consumer behavior, demand patterns, and sales trends, businesses can adjust their strategies accordingly. Retailers can use retail predictive analytics to plan product launches, sales promotions, and even marketing campaigns with a higher degree of certainty.
For example, predictive models can help retailers determine the optimal time for a product launch based on past sales data and seasonal trends. This reduces the risk of underperforming product launches and ensures that marketing campaigns align with customer demand.
Examples of Successful Retailers Using Predictive Insights to Shape Business Strategies
Several major retailers have successfully implemented retail predictive analytics to inform their business strategies. For instance, companies like Amazon and Target use predictive insights to personalize their marketing and optimize their inventory management. These retailers rely heavily on retail predictive analytics to forecast demand, adjust their pricing strategies, and plan targeted marketing campaigns that resonate with their customers.
Retail analytics also informs strategic decisions around store expansion, merchandising, and even partnerships. By using data to predict future market trends, retailers can make more informed decisions that drive long-term success.
Impact on Inventory Management, Marketing, and Sales
Retail predictive analytics has a profound impact on inventory management, marketing, and sales strategies. In inventory management, predictive models help businesses optimize stock levels, reduce waste, and ensure product availability without overstocking. In marketing, retail analytics enables businesses to deliver targeted campaigns based on customer preferences, boosting engagement and conversion rates. Lastly, sales strategies benefit from predictive insights by enabling retailers to forecast sales trends and adjust their efforts accordingly.
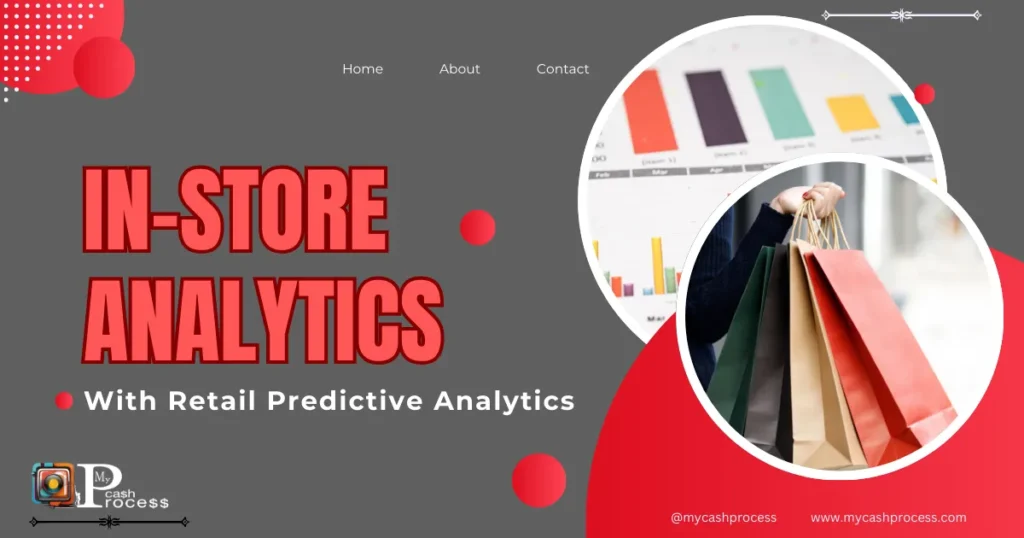
Implementing Retail Predictive Analytics in Your Store
Integrating retail predictive analytics into retail operations requires careful planning, investment in technology, and a clear strategy for data collection and analysis. However, the benefits of adopting predictive analytics far outweigh the initial setup.
Steps to Integrate Predictive Analytics Tools into Your Retail Operations
- Assess Your Current Data Infrastructure: Before implementing retail predictive analytics, it is crucial to assess your current data systems. Ensure that your data collection methods are accurate, efficient, and capable of handling the volume of data required for predictive modeling.
- Choose the Right Predictive Analytics Tools: Select tools that suit your business size and objectives. Some businesses may benefit from sophisticated AI-powered platforms, while others may require more basic analytics software.
- Train Your Team: Predictive analytics tools can be complex, so training your team is essential. Ensure that employees understand how to use these tools effectively and interpret the data to make informed decisions.
- Start with a Pilot Program: To minimize risks, consider starting with a pilot program to test predictive analytics in a limited area of your store. This will allow you to measure the effectiveness of the tools before rolling them out on a larger scale.
- Continuously Monitor and Optimize: Predictive analytics is not a one-time solution. Continuously monitor the performance of your models and adjust them as necessary to ensure that they remain accurate and relevant.
Key Considerations for Adopting New Technologies
When adopting retail predictive analytics tools, it is essential to consider several factors:
- Data Security: Ensure that your customer and business data is secure and protected against breaches.
- Integration with Existing Systems: Choose tools that integrate well with your current systems to ensure seamless data flow.
- Scalability: Ensure that your predictive analytics tools can scale with your business as it grows.
Overcoming Common Challenges When Implementing Retail Analytics
Some common challenges when implementing retail predictive analytics include:
- Data Quality Issues: Inaccurate or incomplete data can lead to faulty predictions. It’s vital to ensure that the data collected is accurate and clean.
- Resistance to Change: Some employees may be resistant to adopting new technologies. Providing adequate training and demonstrating the value of predictive analytics can help overcome this hurdle.
- Cost and Complexity: The initial investment in predictive analytics tools can be costly. However, the return on investment (ROI) from improved efficiency and decision-making often justifies the expense.
Future Trends in Retail Predictive Analytics
As technology evolves, so too will the capabilities of retail predictive analytics. Emerging technologies, such as AI and machine learning, will continue to enhance the accuracy and scope of predictive models.
Emerging Technologies and Innovations in Predictive Analytics
AI, machine learning, and natural language processing (NLP) are paving the way for even more sophisticated retail predictive analytics models. These technologies will allow retailers to analyze even more complex datasets, making predictions with greater accuracy.
For example, AI can analyze unstructured data from social media, customer reviews, and even video footage to predict future trends. These innovations will significantly expand the capabilities of predictive analytics, offering retailers even more detailed and actionable insights.
How AI and Machine Learning Will Further Enhance Predictive Models
AI and machine learning will enable retail predictive analytics to evolve into more autonomous systems. These systems will continuously learn from new data, improving their accuracy over time. By integrating these technologies into their analytics strategies, retailers can gain deeper insights into customer behavior, making their predictions even more precise.
Predictions for the Future of Retail Predictive Analytics
As AI, machine learning, and big data continue to advance, retail predictive analytics will become even more integral to the retail industry. In the future, retailers will be able to predict consumer preferences with near-perfect accuracy, allowing for hyper-personalized marketing, smarter inventory management, and even more efficient store operations.
Leveraging Data Analytics for Retail to Drive Personalization and Engagement
Personalization is key to driving customer engagement and loyalty in retail. By leveraging data and predictive models, businesses can create highly personalized experiences that resonate with individual customers. Predictive analytics allows retailers to better understand customer preferences, habits, and purchase history, enabling them to tailor their offers, messaging, and product recommendations.
Using Predictive Analytics for Targeted Marketing
Predictive analytics plays a crucial role in enabling data analytics to drive more targeted marketing campaigns. By analyzing customer behaviors and transaction histories, businesses can predict the types of products or services a customer is most likely to purchase. This allows retailers to deliver personalized offers, discounts, and product recommendations to the right customers at the right time.
For instance, a clothing retailer can use predictive analytics to identify customers who are likely to purchase specific seasonal items based on previous purchasing patterns. With data analytics, they can then create targeted email campaigns, offering personalized discounts or product bundles to these customers, significantly improving engagement and conversion rates.
Optimizing Customer Segmentation Using Predictive Analytics
Another way predictive analytics supports data analytics is through more effective customer segmentation. By segmenting customers based on their preferences, behavior, and purchase patterns, retailers can create highly targeted marketing strategies that appeal to each group’s unique needs. This level of segmentation allows businesses to increase the relevance of their communications, boosting customer engagement and satisfaction.
For example, predictive analytics could help identify high-value customers who tend to make frequent, high-ticket purchases. By leveraging data analytics, these customers could be targeted with loyalty rewards or exclusive offers, reinforcing their relationship with the brand and encouraging repeat purchases.
Improving the In-Store Experience with Data Analytics
Predictive analytics is not only valuable for digital engagement but also for enhancing the in-store customer experience. Using data analytics, stores can understand foot traffic patterns, product preferences, and dwell times within the store. This data can then be used to optimize store layouts, product placements, and staffing levels to ensure customers have the best possible experience when shopping in person.
For instance, if data analytics shows that certain products are frequently purchased together, retailers can adjust their in-store displays to place these products in close proximity. Additionally, predictive analytics can help determine the ideal times for product restocking and sales staffing to create a seamless in-store experience that maximizes customer satisfaction.
Leveraging In Store Analytics for Real-Time Retail Success
In the world of modern retail, in store analytics is one of the most powerful tools available to drive immediate improvements in customer experience, sales performance, and overall operational efficiency. By utilizing in-store analytics, retailers can access real-time data that reveals consumer behaviors, purchasing patterns, and store traffic. This section will explore how in store analytics plays a crucial role in enhancing retail predictive analytics.
How In Store Analytics Improves Customer Engagement
- In-store analytics allows retailers to track customer movements and interactions in real time, helping identify popular products and optimal store layouts.
- By analyzing foot traffic and dwell times in specific areas, businesses can refine their strategies for product placement and promotions.
Operational Efficiency Boosted by In Store Analytics
- Using in store analytics, stores can track inventory levels in real time, reducing the risk of stockouts or overstocking, which leads to better inventory management.
- The data gathered through in store analytics can help optimize staff scheduling, ensuring that the right number of employees are available during peak hours.
Real-Time Data for Smarter Decision Making
- In store analytics provides valuable insights that allow store managers to adjust their strategies immediately.
- Retailers can make on-the-fly adjustments based on in-store data, such as repositioning products to increase visibility or updating promotions based on customer preferences.
Impact of In Store Analytics on Sales Growth
- By leveraging in store analytics, retailers can identify trends that directly influence sales growth, such as identifying which products are most frequently picked up but not purchased.
- Additionally, in store analytics can pinpoint areas of the store where customers are spending the most time, indicating high-demand products or services that should be prioritized.
Conclusion
The role of retail predictive analytics in the retail industry is undeniable. By leveraging data to forecast trends, optimize operations, and enhance the customer experience, businesses can unlock significant competitive advantages. From retail store analysis reports to in-depth insights on customer behavior, data analytics for retail is transforming how businesses operate.
Adopting retail predictive analytics is no longer a luxury but a necessity for retail success. Retailers who implement predictive tools are better equipped to make data-driven decisions, anticipate market changes, and enhance the overall customer journey. As the future of retail evolves, embracing predictive analytics will be key to staying ahead of the competition.
Warning
While retail predictive analytics offers significant benefits, relying too heavily on predictions without considering other factors can be risky. Predictive models are based on historical data, and unexpected market changes or new consumer behaviors can lead to inaccurate predictions. It’s essential to balance predictive insights with human judgment and adaptability to ensure a holistic approach to decision-making.
Follow us on Pinterest, Twitter X, Facebook, Instagram, Quora, TikTok, Discord, YouTube, and WhatsApp Channel.
Advice
To successfully integrate retail predictive analytics into your operations:
- Start small with a pilot program.
- Ensure that your data quality is top-notch.
- Train your team to understand and leverage predictive tools effectively.
- Continuously monitor and adjust your predictive models to maintain accuracy.
FAQs
-
What is retail predictive analytics?
It involves using data and predictive models to forecast future trends, customer behavior, and business outcomes in retail.
-
How does predictive analytics help retailers improve customer experience?
By analyzing customer behavior and trends, predictive analytics allows retailers to personalize shopping experiences and optimize store layouts.
-
What technologies are used in retail predictive analytics?
AI, machine learning, and big data technologies are commonly used to enhance predictive models in retail.
-
Can predictive analytics help with inventory management?
Yes, predictive analytics helps retailers forecast demand and optimize inventory levels, reducing overstocking or stockouts.