Introduction
In today’s fast-paced retail environment, staying ahead of the competition and understanding consumer behavior is more important than ever. As traditional methods of tracking store performance become less effective, the need for data analytics for retail has grown significantly. Retailers are increasingly turning to retail analytics to gather meaningful insights and optimize their operations. Through data analytics for retail, businesses can unlock new opportunities to improve customer experience, enhance decision-making, and boost sales.
The importance of data analytics for retail cannot be overstated. By leveraging the power of analytics, retailers can better understand the patterns that drive sales, anticipate customer needs, and improve the effectiveness of marketing strategies. Retail store analysis report, retail predictive analytics, and in store analytics are all powerful tools that contribute to a more data-driven approach in retail.
In this article, we will explore how data analytics for retail can revolutionize store performance, and how retailers can harness these tools to drive growth. We will dive into the concepts behind retail analytics, discuss practical applications such as in store analytics, and look at the role of retail predictive analytics in shaping business strategies. By the end, you will have a clear understanding of how these data-driven insights can transform your retail operations.
Quick Access Guide
What is Data Analytics for Retail?
Definition and Core Concepts of Data Analytics for Retail
At its core, data analytics for retail refers to the process of collecting, analyzing, and interpreting data to gain insights into retail operations and consumer behavior. This involves examining various data points, such as sales figures, customer demographics, transaction history, and even store layout effectiveness. Through advanced algorithms and statistical techniques, data analytics for retail allows businesses to make informed decisions, which can enhance everything from inventory management to marketing strategies.
The key objective of data analytics for retail is to extract actionable insights from data, turning raw numbers into meaningful information that can drive business decisions. Retailers can leverage this data to understand customer preferences, predict demand, optimize inventory, and personalize marketing efforts. With retail store analysis report, businesses can evaluate the effectiveness of their operations and identify areas of improvement.
Purpose of Using Data Analytics for Retail in Enhancing Store Performance
The primary goal of data analytics for retail is to enhance store performance by improving various aspects of the business. For instance, by utilizing retail analytics, businesses can pinpoint underperforming products or categories and make adjustments to optimize sales. In store analytics can also provide insights into customer behavior, allowing retailers to refine store layouts, product placement, and overall shopping experience. Furthermore, retail predictive analytics helps forecast future trends, enabling businesses to plan for changes in customer demand.
In addition to improving store operations, data analytics for retail can help businesses streamline their marketing efforts. By analyzing customer purchasing patterns, retailers can create targeted campaigns that speak directly to their audience, increasing the likelihood of conversions. This type of data-driven approach allows businesses to allocate marketing resources more effectively and achieve a higher return on investment (ROI).
How Retail Analytics Supports Decision-Making
Retail analytics empowers business leaders to make more informed decisions. Rather than relying on intuition or guesswork, retailers can now base their decisions on concrete data. For example, retail store analysis report can provide insight into which products are the most profitable, which customer segments generate the highest sales, and which areas of the store are most frequently visited. These insights can help managers make smarter decisions regarding inventory management, product pricing, and staffing.
The use of data analytics for retail also enhances real-time decision-making. In today’s competitive market, it’s essential for retailers to be able to quickly adjust to shifts in consumer behavior. With access to up-to-date data, businesses can adapt to changes in demand, stock levels, and sales trends. Retail predictive analytics can even provide forecasting models that allow businesses to prepare for future changes in the market, ensuring they stay one step ahead of the competition.
The Power of Retail Analytics in Transforming Store Operations
Key Areas Where Retail Analytics Can Impact Store Operations
Retail analytics has the power to improve various aspects of store operations, leading to enhanced efficiency and profitability. One of the key areas where retail analytics makes an impact is in inventory management. By analyzing sales data and trends, retailers can optimize stock levels and reduce the risk of overstocking or stockouts. This is especially important for retailers who deal with seasonal or fast-moving products, as data analytics can help predict demand fluctuations.
Another area that benefits from retail analytics is staffing. Through the analysis of foot traffic and sales data, retailers can determine the optimal number of employees needed at different times of the day or week. This ensures that stores are properly staffed, improving customer service while reducing labor costs. In store analytics also helps managers identify which store sections receive the most foot traffic, allowing them to allocate resources more efficiently.
The Role of In Store Analytics in Understanding Customer Behavior
In store analytics plays a crucial role in understanding how customers interact with the physical store environment. By tracking customer movements, dwell times, and product interactions, retailers can gain valuable insights into shopping behaviors. For instance, in store analytics can reveal which areas of the store attract the most attention, helping retailers optimize store layout and product placement.
Moreover, in store analytics provides data on customer preferences and buying patterns. By understanding which products customers are most likely to purchase or spend time interacting with, retailers can tailor their offerings to better meet customer needs. This data-driven approach to store management helps create a more personalized shopping experience, which can lead to higher customer satisfaction and increased sales.
Benefits of Integrating Retail Store Analysis Report into Everyday Operations
Integrating retail store analysis report into daily operations allows retailers to continuously monitor and optimize their performance. With detailed insights into sales trends, customer behavior, and operational efficiency, businesses can identify areas for improvement and take immediate action. For example, if a certain product is consistently underperforming, a retailer can use retail analytics to determine if the issue lies in pricing, placement, or customer demand.
Another benefit of using retail store analysis report is the ability to track and measure the impact of marketing campaigns. Retailers can assess the effectiveness of their promotions by analyzing sales data before, during, and after the campaign. This allows businesses to fine-tune their marketing strategies, ensuring they invest in initiatives that generate the highest return on investment.
Enhancing Customer Experience Through Data Analytics for Retail and Retail Predictive Analytics
How Data Analytics for Retail Can Personalize the Customer Experience
One of the most compelling benefits of data analytics for retail is its ability to personalize the customer experience. In today’s competitive retail environment, customers expect a tailored experience that speaks directly to their preferences and needs. By leveraging retail analytics, retailers can gather detailed insights into consumer behavior, such as purchasing patterns, browsing habits, and product preferences.
With these insights, retailers can offer personalized recommendations, discounts, and promotions based on individual customer profiles. For example, an online retailer can use retail predictive analytics to recommend products similar to what a customer has purchased or viewed in the past. In physical stores, in store analytics can help identify customers’ most frequently visited sections and product categories, enabling store associates to make personalized suggestions during their visit.
By integrating data analytics for retail into the customer journey, retailers can create an engaging and customized shopping experience that not only enhances satisfaction but also drives loyalty. Personalized experiences have been shown to increase customer retention rates, improve lifetime value, and boost sales. This approach empowers retailers to meet customer expectations while differentiating themselves from competitors.
The Role of Retail Predictive Analytics in Anticipating Customer Needs
Retail predictive analytics is another powerful tool that enables retailers to stay ahead of customer demand. By analyzing historical data and using advanced algorithms, predictive models can forecast future trends, customer preferences, and purchasing behaviors. This allows retailers to anticipate customer needs before they arise, ensuring they have the right products available at the right time.
For example, retail predictive analytics can help businesses identify seasonal demand fluctuations, ensuring they are stocked with popular products before peak seasons. It can also forecast shifts in customer preferences, helping retailers stay relevant by adjusting their product offerings accordingly. By anticipating demand in advance, retailers can improve inventory management, reduce the risk of stockouts, and avoid overstocking slow-moving products.
Real-life examples of retail predictive analytics in action include personalized marketing campaigns based on predicted customer behavior. Retailers can use these insights to target customers with tailored offers, promotions, or discounts, increasing the likelihood of conversions and enhancing the customer experience.
Optimizing Product Placement and Store Layout with In Store Analytics and Retail Analytics
Importance of In Store Analytics in Optimizing Store Layout
In store analytics provides valuable insights into how customers interact with a physical store’s layout. By tracking customer movements and dwell times within specific areas of the store, retailers can identify which sections of the store attract the most attention and which are underperforming. This information is crucial for optimizing store layouts to improve the flow of traffic and highlight high-margin products.
For example, in store analytics can reveal if customers tend to spend more time in certain aisles or product categories, while other sections are bypassed. With this knowledge, retailers can adjust product placement, rearrange displays, or add signage to draw attention to areas that are underperforming. By strategically placing high-demand products near high-traffic areas, businesses can maximize sales and enhance the shopping experience.
Using Retail Store Analysis Report to Enhance Product Placement Strategies
Integrating retail store analysis reports into the decision-making process can further optimize product placement strategies. This report offers a detailed breakdown of sales data, customer demographics, and buying patterns, enabling retailers to assess which products resonate most with specific customer segments. Using this data, businesses can position their best-selling products in prime locations to increase visibility and drive sales.
By combining in store analytics with retail store analysis report, retailers can create a data-driven approach to product placement that maximizes revenue potential. For instance, products that are frequently purchased together can be placed near each other, encouraging cross-selling opportunities and increasing average order value.
Examples of Effective Product Placement Influenced by Retail Analytics
Successful product placement strategies driven by retail analytics can significantly boost sales. One example is a grocery store that uses in store analytics to monitor customer behavior. By analyzing foot traffic and purchase patterns, the store determines that customers who buy bread often purchase butter. The store can then place these products next to each other, making it easier for customers to complete their purchase and increasing sales of both items.
Another example comes from the fashion industry, where retail store analysis report helps identify popular clothing items. By positioning high-demand products in the store’s front window or near the checkout area, retailers can capitalize on impulse buying and increase sales of those items.
How Retail Predictive Analytics Shapes Business Strategy
Overview of Retail Predictive Analytics and Its Forecasting Capabilities
Retail predictive analytics uses historical data, statistical algorithms, and machine learning models to forecast future outcomes. In the context of retail, it helps predict customer demand, sales trends, and inventory needs. By leveraging these insights, retailers can optimize inventory levels, plan promotions, and develop more effective marketing strategies.
The core benefit of retail predictive analytics is its ability to anticipate changes in the market. For instance, retailers can predict when demand for a product will spike due to seasonal factors or emerging trends. By having this foresight, businesses can ensure they are prepared to meet customer demand without overstocking or understocking products.
How Predictive Models Help Retailers Anticipate Future Trends and Demand
With retail predictive analytics, businesses can anticipate shifts in consumer behavior and adapt their strategies accordingly. For example, if predictive models indicate that a certain product category is gaining popularity, retailers can increase their inventory and adjust their marketing efforts to capitalize on this trend. This proactive approach helps retailers stay ahead of competitors and meet customer expectations.
Predictive models can also identify emerging trends before they become mainstream, giving retailers a competitive edge. For instance, if data analytics show that a particular fashion style is gaining traction, retailers can adjust their product assortment and marketing strategies to target the growing demand.
Real-Life Examples of Successful Implementations of Retail Predictive Analytics
A notable example of successful retail predictive analytics implementation comes from the fashion industry. Retailers have used predictive models to forecast the demand for certain styles and colors based on past sales data, weather patterns, and social media trends. By accurately predicting demand, these retailers have been able to optimize their inventory and avoid the risk of stockouts or overstocking.
Another example is in the grocery industry, where retail predictive analytics has been used to forecast demand for perishable items. Retailers use predictive models to ensure that they have the right amount of fresh produce in stock, reducing food waste and increasing customer satisfaction by providing fresher products.
The Benefits of In Store Analytics in Offline Retail
Why Offline Retailers Need In Store Analytics for Better Customer Insights
For offline retailers, in store analytics is essential for understanding how customers interact with the physical store environment. Traditional methods of gathering feedback, such as surveys or manual observation, often fail to provide a comprehensive understanding of customer behavior. In store analytics fills this gap by offering real-time, objective data on how customers move through the store, what products they engage with, and which areas they spend the most time in.
By leveraging in store analytics, offline retailers can gather insights that help optimize the shopping experience. For instance, if in store analytics shows that customers are spending more time near the entrance but not engaging with the products further inside, the store layout can be adjusted to guide customers deeper into the store. This leads to increased engagement with products, ultimately boosting sales.
How In Store Analytics Improves the In-Store Shopping Experience
In store analytics plays a vital role in improving the in-store shopping experience. By tracking customer behavior, retailers can identify pain points, such as areas where customers feel frustrated or confused. This allows store managers to make data-driven adjustments that enhance the shopping experience. For example, if customers tend to linger in one section of the store due to unclear signage, retailers can improve the layout and signage to make the navigation process smoother.
Additionally, in store analytics can help retailers create targeted marketing campaigns based on customer behavior. For instance, if the analytics show that a particular product is frequently viewed but not purchased, a retailer might offer a limited-time discount or promotion to encourage customers to complete their purchase.
Key Metrics Tracked Through In Store Analytics to Boost Sales
In store analytics tracks several key metrics that can help boost sales and improve store performance. These metrics include foot traffic, dwell time, conversion rates, and product engagement. By analyzing these data points, retailers can better understand customer preferences and optimize store operations.
For instance, tracking dwell time can reveal which products capture customers’ attention the longest. If certain products have higher dwell times but lower conversion rates, it may indicate that customers are interested in the product but need more convincing. Retailers can then use this information to improve product placement, display techniques, or pricing strategies to encourage purchases.
Leveraging Customer Feedback for Store Optimization
Understanding the Importance of Customer Feedback
Customer feedback has always been an integral part of improving retail operations, but with the advent of data analytics, its importance has grown exponentially. Today, customer feedback provides valuable insights into customer preferences, behaviors, and pain points, enabling retailers to fine-tune their operations for maximum success.
Feedback allows stores to identify which products or services are most favored by their customers and which may need adjustment. Moreover, understanding customer sentiment helps in making informed decisions regarding store layout, product pricing, and marketing efforts. Ignoring customer feedback may lead to missed opportunities and a decline in customer loyalty.
Methods of Gathering Customer Feedback
Retailers can gather customer feedback through various channels, each offering different types of insights. Common methods include:
- In-store surveys: Simple surveys or questionnaires offered to customers while they shop can provide instant feedback about their experience.
- Social media monitoring: With the rise of social media platforms, retailers can track what customers are saying online, providing a candid perspective on customer sentiment.
- Post-purchase reviews: After a customer makes a purchase, retailers can request reviews through email, online forms, or their website. These reviews help assess product satisfaction and areas for improvement.
- Loyalty program insights: When customers sign up for loyalty programs, their interactions with the store—whether online or in-store—can help identify preferences, purchase patterns, and overall satisfaction.
Each of these methods offers unique advantages and when combined, they provide a comprehensive view of customer opinions and preferences.
How Customer Feedback Enhances Retail Strategies
By incorporating customer feedback, retailers can optimize many aspects of their business. Some of the areas where customer insights directly influence retail strategies include:
- Product Selection: Customer feedback reveals what customers want and what they dislike. Retailers can adjust their inventory accordingly, discontinuing underperforming products and stocking items that are more in demand.
- Store Layout: Feedback can help retailers make informed decisions about store layout. If customers complain about crowded aisles or difficulty finding products, these insights can lead to improved store navigation and better product placement.
- Sales Tactics: Understanding customer pain points and desires allows retailers to create more personalized and effective sales tactics. For instance, knowing when and how customers prefer to receive discounts can optimize promotional strategies.
- Customer Retention: Feedback provides an opportunity to connect with customers on a personal level. When retailers respond to feedback and make improvements based on customer suggestions, it fosters a deeper sense of loyalty and trust.
In summary, feedback not only helps retailers meet their customers’ needs but also guides them in creating a better overall shopping experience, driving higher satisfaction and sales.
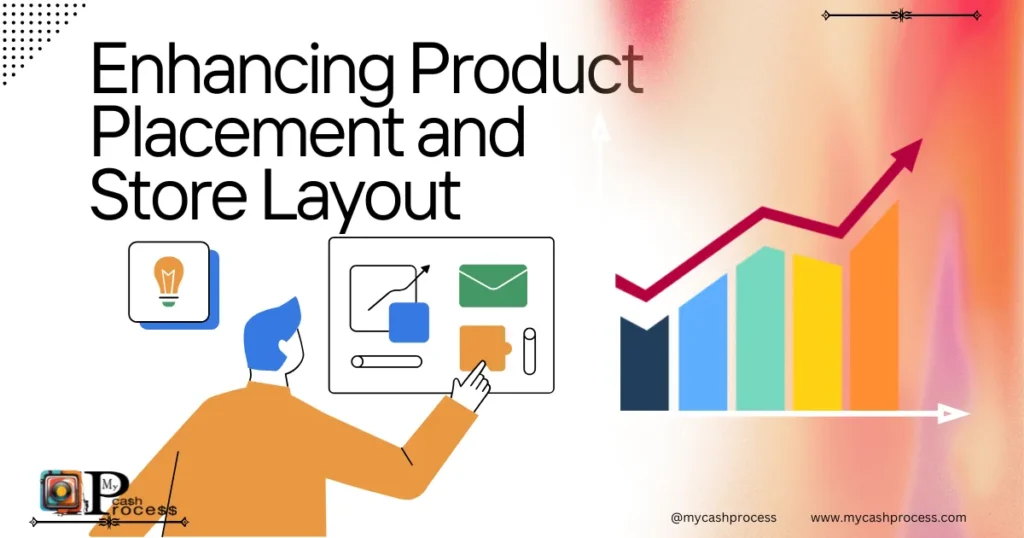
Real-Time Analytics and Its Impact on Decision-Making
The Power of Real-Time Data in Retail
Real-time data analytics has revolutionized the way retailers make decisions. With live data coming in from various touchpoints like sales transactions, inventory levels, and customer behaviors, retailers now have the power to make immediate, data-driven decisions.
Real-time data helps businesses stay agile, reacting promptly to changes in the market or consumer behavior. Unlike traditional reporting, which is static and periodic, real-time analytics gives businesses a continuous stream of actionable insights, enabling them to adapt quickly to fluctuations and capitalize on opportunities as they arise.
Using Real-Time Analytics to Manage Store Operations
Real-time analytics offers several practical applications for improving store operations. Some of the key benefits include:
- Inventory Management: With real-time data on stock levels, retailers can ensure that inventory is replenished automatically, avoiding stockouts or overstock situations. Additionally, they can monitor sales trends to determine which products are flying off the shelves and which need a promotional boost.
- Sales Adjustments: During peak shopping times, real-time analytics can be used to adjust staffing levels, optimizing labor costs without sacrificing customer service. Additionally, sales strategies can be adapted based on immediate customer behavior, such as pushing specific products that are currently trending.
- Customer Behavior Insights: Real-time analytics allows retailers to monitor in-store customer activity. If a customer spends more time in one section of the store, analytics can reveal what products they are drawn to, providing opportunities for better product placement and promotions.
- Dynamic Pricing: By analyzing real-time market data, retailers can adjust pricing strategies in response to supply and demand, competitor pricing, or customer purchasing patterns. This ensures that the store remains competitive and maximizes profitability.
Case Studies of Real-Time Analytics in Retail
Several well-known retailers have successfully implemented real-time analytics to enhance their operations. For example:
- Walmart uses real-time data to track inventory and forecast demand across its vast network of stores, ensuring products are stocked and available for customers when they need them.
- Zara is another example where real-time analytics helps the company stay ahead of fashion trends. Their ability to react quickly to customer preferences through real-time sales and inventory tracking gives them a significant competitive advantage.
These case studies highlight the tremendous potential of real-time analytics to transform retail operations, drive growth, and ensure customer satisfaction.
The Role of Employee Training in Maximizing Data Utilization
Why Employee Training Is Key to Successful Data Integration
As retail analytics tools become more advanced, the need for well-trained employees who can effectively interpret and act on data has never been greater. Without the proper training, data might go underutilized, and insights may be lost in the noise.
Employee training plays a critical role in ensuring that everyone in the organization understands how to use data analytics tools and apply them to improve store performance. This includes training staff at all levels—from sales associates who use mobile analytics to optimize customer service, to managers who analyze sales trends and inventory patterns to make business decisions.
When employees are well-versed in data analytics, they can more effectively leverage these tools to drive decisions that contribute to higher efficiency and profitability.
Best Practices for Training Employees on Data Analytics Tools
To ensure that employees gain the necessary skills to utilize data analytics tools, retailers should implement the following best practices:
- Tailored Training Programs: Develop training materials tailored to the specific needs of each department. For instance, sales associates may require training on customer behavior analysis, while managers need to focus on inventory forecasting and pricing strategies.
- Hands-On Training: Provide employees with opportunities to engage with real data through simulations or practical applications, enabling them to learn by doing. This ensures that employees are comfortable with the tools they will be using.
- Regular Training Updates: Data analytics tools evolve rapidly, so it’s crucial to offer continuous training to ensure employees are up to date with the latest features and techniques.
- Encourage a Data-Driven Culture: Foster a culture that prioritizes data-driven decision-making. When employees see the value of data in improving their work, they are more likely to embrace and utilize the analytics tools available to them.
How Data-Literate Employees Can Improve Retail Efficiency
Data-literate employees can make a significant impact on the overall efficiency of a retail business. When staff members are trained to understand and use analytics, they become more proactive in their roles:
- Inventory and Stock Optimization: Employees can make more informed decisions about restocking items, ensuring that high-demand products are always available while minimizing excess inventory of slower-moving goods.
- Customer Service Improvements: Sales associates who understand customer purchase patterns can better recommend products, anticipate customer needs, and provide a more personalized shopping experience.
- Operational Efficiencies: Managers who are data-literate can identify bottlenecks, inefficiencies, or areas for cost savings. For instance, understanding foot traffic data allows managers to optimize staff schedules during peak hours.
Data-literate employees ultimately create a more responsive, efficient, and customer-focused environment, benefiting both the store and its customers.
The Integration of E-commerce and Physical Stores
Blending Online and In-Store Shopping Experiences
As consumer behavior continues to evolve, the lines between online and in-store shopping are becoming increasingly blurred. Many customers now expect to have a seamless shopping experience that spans both channels. Whether they are browsing online or visiting a physical store, they want consistent pricing, availability, and service.
Integrating e-commerce with physical stores creates a unified experience that increases customer satisfaction and drives sales. This approach is known as omnichannel retailing, and it allows businesses to meet customers where they are—whether online, in-store, or even through social media.
How E-commerce Integration Enhances Retail Operations
By integrating e-commerce with physical stores, retailers can streamline their operations in several ways:
- Inventory Visibility: Online and in-store stock levels are updated in real time, making it easier to manage inventory across multiple sales channels. This eliminates the risk of overstocking or running out of popular products.
- Click-and-Collect Services: Many retailers are offering the option for customers to order products online and pick them up in-store. This service not only provides convenience for customers but also drives foot traffic to the store.
- Seamless Customer Service: Whether shopping online or in-store, customers can access the same high level of customer service. Retailers can ensure that returns and exchanges are simple and consistent across both channels, reducing frustration for customers.
- Personalization: Retailers can collect data from both online and in-store interactions to create personalized shopping experiences for customers. This might include personalized product recommendations or special promotions tailored to individual preferences.
The integration of e-commerce and physical stores is no longer just a trend—it’s becoming a necessity for retailers who want to stay competitive in the evolving marketplace.
The Future of Retail Technology: Innovations to Watch
The Role of AI and Machine Learning in Retail
Artificial intelligence (AI) and machine learning (ML) are transforming the retail industry in profound ways. From enhancing the customer experience to optimizing operations, these technologies are making it easier for retailers to predict trends, automate tasks, and personalize offerings.
AI can be used to predict customer purchasing behaviors by analyzing past buying patterns, allowing retailers to stock the right products at the right time. Machine learning algorithms can also help with dynamic pricing, adjusting prices based on factors such as demand, competition, and customer profiles.
Emerging Technologies Shaping the Retail Landscape
Retailers should keep an eye on the following technologies as they evolve:
- Augmented Reality (AR): AR is transforming the shopping experience by allowing customers to virtually try on clothes, visualize products in their home environment, or experience virtual store tours. It enhances the in-store experience and can even be integrated with mobile shopping apps.
- Smart Shelves: Using IoT (Internet of Things) sensors, smart shelves can monitor product levels in real-time and automatically alert staff when stock is low. This technology improves inventory management and ensures that customers can always find the products they’re looking for.
- Automated Checkout: As retailers look for ways to streamline operations and enhance customer convenience, automated checkout systems are becoming more common. These systems use computer vision and sensors to allow customers to scan and pay for their items without the need for traditional checkout counters.
- Robotic Process Automation (RPA): RPA can help automate back-office tasks, such as processing inventory orders or managing logistics. This increases efficiency and allows employees to focus on higher-value activities, such as customer service and sales strategy.
These technologies will continue to evolve, offering retailers innovative solutions to meet the changing demands of consumers and improve operational efficiency.
Enhancing Customer Loyalty with Reward Programs
Building Long-Term Relationships with Customers
Customer loyalty is vital to the success of any retail business. Loyal customers tend to spend more, return more frequently, and advocate for your brand. One of the most effective ways to build customer loyalty is through a well-designed reward program.
Reward programs incentivize customers to keep coming back by offering them perks such as discounts, exclusive offers, and early access to new products. These programs create an emotional connection with customers, making them feel valued and appreciated.
Types of Reward Programs and How They Work
There are several types of reward programs, each catering to different business needs and customer preferences:
- Points-Based Programs: Customers earn points for every purchase they make, which can then be redeemed for discounts, products, or other rewards. This type of program encourages repeat business and rewards customers for their loyalty.
- Tiered Programs: In a tiered reward program, customers achieve different status levels based on their spending or activity. Higher tiers unlock more exclusive benefits, motivating customers to increase their engagement and spend more.
- Referral Programs: Many retailers offer rewards to customers who refer friends or family members to the business. This creates an organic way to expand the customer base while rewarding loyal customers for their efforts.
- Subscription-Based Programs: Some retailers offer subscription services where customers pay a monthly fee to receive ongoing benefits, such as discounts, free shipping, or access to exclusive products. This model creates recurring revenue and encourages customer retention.
How Reward Programs Increase Customer Retention
A well-executed reward program can boost customer retention in several ways:
- Increased Spending: The more a customer engages with your reward program, the more they are likely to spend in order to reach the next reward level or redeem their points.
- Customer Engagement: Reward programs keep customers engaged with your brand by offering regular incentives for their continued loyalty. This keeps your brand top of mind.
- Personalized Offers: By collecting data through your reward program, you can offer personalized rewards based on a customer’s preferences and purchase history, making them feel valued and enhancing their shopping experience.
Reward programs not only foster customer loyalty but also serve as a valuable tool for gathering insights into customer behavior, which can be used to optimize your marketing and sales strategies.
Conclusion
In conclusion, data analytics for retail is a powerful tool that offers numerous benefits for businesses seeking to enhance store performance, improve customer experiences, and drive growth. From retail predictive analytics that help forecast demand to in store analytics that optimize store layouts, the potential for retail analytics is immense.
As retail continues to evolve, embracing data analytics for retail will be essential for staying competitive in a rapidly changing market. Retailers who leverage these insights will not only improve operational efficiency but will also be better positioned to anticipate trends, personalize the customer experience, and optimize their marketing strategies.
Warning
Retailers who neglect to adopt data analytics for retail risk falling behind in an increasingly data-driven world. The competition is embracing analytics, and businesses that fail to keep up may struggle to make informed decisions or miss out on valuable opportunities.
Furthermore, handling customer and sales data comes with risks, such as potential data breaches. Retailers must ensure they follow best practices for data security to protect sensitive information.
Follow us on Pinterest, Twitter X, Facebook, Instagram, Quora, TikTok, Discord, YouTube, and WhatsApp Channel.
Advice
Retailers should begin by identifying key areas where data analytics for retail can have the most impact. Starting small, such as implementing in store analytics or generating a retail store analysis report, can provide valuable insights that lead to actionable improvements. As businesses become more comfortable with analytics tools, they can expand their use to predictive models and more advanced applications, staying ahead of trends and maintaining a competitive edge.
FAQs:
-
What is data analytics for retail?
Data analytics for retail refers to the use of data analysis techniques to extract insights from retail data. These insights help retailers understand customer behavior, optimize operations, and make data-driven decisions to improve sales, inventory management, and customer satisfaction.
-
How can data analytics improve the customer experience in retail?
By analyzing customer behavior and preferences, data analytics allows retailers to offer personalized recommendations, promotions, and tailored shopping experiences. This enhances customer satisfaction, increases loyalty, and ultimately drives sales.
-
What is Retail predictive analytics?
Retail predictive analytics uses historical data and machine learning algorithms to forecast future trends and customer behavior. This helps retailers anticipate demand, plan inventory, optimize marketing strategies, and improve overall operational efficiency.
-
How does in store analytics work?
In store analytics involves tracking customer movements within a physical store to gather insights on foot traffic, product engagement, and dwell times. This data helps retailers optimize store layouts, improve product placement, and enhance the overall shopping experience.
-
What are the key metrics tracked in store analytics?
Key metrics tracked in-store analytics include foot traffic, dwell time, conversion rates, product engagement, and purchase behavior. Analyzing these metrics allows retailers to understand customer preferences and make data-driven decisions to improve store performance.