Introduction
In the dynamic world of retail, understanding customer behavior and optimizing store performance is crucial to success. With the advent of data-driven decision-making, retail analytics has become the backbone of efficient business operations. Retailers who embrace this strategic approach can gain valuable insights into sales trends, customer preferences, and inventory management. By leveraging retail analytics, businesses can make informed decisions that lead to improved profitability, enhanced customer experience, and optimized operational efficiency.
In this article, we will explore how retail analytics can be a game-changer for your retail business. We’ll discuss the essential components of retail analytics, how retail predictive analytics helps forecast trends, and how in-store analytics provides insights into customer behavior. Additionally, we’ll delve into the value of retail store analysis reports, and how integrating data analytics into retail operations can maximize return on investment (ROI).
Quick Access Guide
Understanding Retail Analytics
Definition of Retail Analytics
Retail analytics refers to the process of collecting, analyzing, and interpreting data to improve retail operations. By focusing on key areas like sales, inventory management, and customer behavior, retail analytics helps businesses uncover insights that drive more effective decision-making. Whether it’s optimizing product placements, streamlining supply chains, or personalizing customer experiences, retail analytics is essential in creating a competitive edge in the marketplace.
Importance of Data-Driven Decisions
The traditional methods of managing retail businesses, such as intuition-based decision-making, are becoming increasingly ineffective. With the influx of data from various sources—point of sale (POS) systems, online platforms, and in-store interactions—data analytics for retail offers a more precise approach. Retailers who adopt data-driven strategies can identify trends, improve operational efficiency, and ultimately increase sales.
Data-driven decision-making is particularly important in identifying inefficiencies. For example, retail predictive analytics can forecast which products are likely to sell out during a particular season or which marketing strategies will generate the best returns. By relying on retail analytics, businesses can confidently make strategic decisions based on facts, not assumptions.
Key Areas Retail Analytics Covers: Sales, Customer Behavior, and Inventory
Retail analytics covers a wide range of areas, but three of the most impactful are:
- Sales Analysis: Understanding which products are selling well and which are underperforming is crucial for making informed decisions. Sales data helps retailers adjust inventory levels and develop targeted marketing strategies.
- Customer Behavior Analysis: By examining how customers interact with products and services—both online and in-store—retailers can personalize their offerings and improve the shopping experience.
- Inventory Management: Retail analytics provides insights into stock levels, demand patterns, and replenishment needs. It helps businesses avoid overstocking or running out of stock, which can lead to lost revenue.
Why Retail Analytics is Crucial for Retail Success
Impact on Decision-Making and Operational Efficiency
Effective decision-making is at the heart of every successful retail business. Retail analytics provides the data required for making these decisions more accurately. With access to real-time data, retailers can quickly respond to market changes, customer preferences, and operational inefficiencies. Whether it’s determining pricing strategies, planning marketing campaigns, or adjusting stock levels, retail analytics allows businesses to stay ahead of the competition.
In addition to decision-making, retail analytics improves operational efficiency by identifying inefficiencies in processes such as supply chain management, labor scheduling, and product stocking. For example, retail predictive analytics helps businesses forecast demand and adjust staffing levels accordingly, ensuring there are enough staff to meet customer needs during peak times.
The Role of Retail Store Analysis Report in Business Success
A retail store analysis report is a critical tool in evaluating the health of a retail operation. By reviewing key performance indicators (KPIs) such as sales, conversion rates, and inventory turnover, businesses can identify strengths and weaknesses in their operations. These reports provide actionable insights that enable retailers to refine their strategies and improve overall performance.
For example, a retail store analysis report can reveal that a particular product is consistently out of stock, suggesting a need for better inventory management. Alternatively, it may show that certain store locations are underperforming, which could prompt a review of the marketing strategy or store layout.
Importance of Accurate Data Collection and Analysis
To ensure that the insights derived from retail analytics are accurate and actionable, it is essential to have robust data collection processes in place. Retailers must gather data from multiple sources, including POS systems, e-commerce platforms, customer feedback, and more. Accurate data collection enables retailers to make informed decisions that are based on a holistic view of their business.
Key Components of Retail Analytics
In-Store Analytics: Gathering and Analyzing In-Store Data
In-store analytics is the process of collecting and analyzing data from physical retail environments. This includes tracking customer movement, dwell times, and purchase behavior within the store. With the help of sensors, cameras, and other tracking technologies, retailers can gather data on how customers interact with products, store layouts, and displays.
By analyzing this data, retailers can gain valuable insights into which areas of the store attract the most attention and which products are being overlooked. This allows them to optimize store layouts, improve product placement, and design more effective in-store promotions.
Retail Predictive Analytics: Forecasting Trends and Customer Needs
Retail predictive analytics uses historical data to forecast future trends and customer behaviors. By analyzing past sales data, weather patterns, and other factors, retailers can predict which products will be in demand during certain periods. This predictive capability helps businesses plan their inventory, marketing strategies, and staffing levels ahead of time.
For example, retail predictive analytics can forecast a surge in demand for outdoor furniture as the summer season approaches, allowing a retailer to stock up on inventory and launch targeted marketing campaigns. This proactive approach ensures that businesses are always prepared for changes in customer demand.
Data Analytics for Retail: Integrating All Data Sources for Comprehensive Insights
Data analytics for retail involves integrating data from multiple sources—such as POS systems, online platforms, and in-store analytics—into one comprehensive system. By combining these various data streams, retailers can gain a 360-degree view of their operations and customer behavior. This integration enables businesses to identify correlations between customer preferences, sales trends, and inventory needs.
For example, by analyzing data from both online and in-store purchases, retailers can identify patterns in customer behavior, such as whether a particular customer prefers to browse products online before making a purchase in-store. These insights can inform cross-channel marketing strategies and improve customer engagement.
Retail In Store Analysis Report: What It Reveals
Key Insights from a Retail Store Analysis Report
A retail store analysis report provides an in-depth look at the performance of a retail business. Key insights include sales trends, customer demographics, inventory turnover, and store traffic patterns. These reports highlight areas of strength and opportunities for improvement.
For example, a retail store analysis report might show that a particular store location has higher foot traffic but lower sales, suggesting that product placement or sales techniques need to be optimized.
How to Interpret Store Performance Metrics
Interpreting store performance metrics is crucial for making informed decisions. Retailers must understand which KPIs are most important for their business and how to measure them effectively. Some key metrics include:
- Sales per square foot: Measures the efficiency of a retail space in generating sales.
- Conversion rate: The percentage of visitors who make a purchase.
- Average transaction value (ATV): The average amount spent per customer.
By analyzing these metrics, retailers can identify areas of improvement and develop strategies to boost sales and profitability.
Utilizing Reports to Drive Store Improvements
The insights from a retail store analysis report can drive continuous improvements. For instance, if the report reveals that certain products are consistently out of stock, the retailer can adjust inventory levels or reorder products more frequently. Similarly, if the report indicates that customers are spending less time in the store, it may be time to rethink the store layout or create more engaging promotions.
Retail Predictive Analytics: Anticipating Future Trends
What Is Retail Predictive Analytics?
Retail predictive analytics is a forward-looking approach that helps retailers predict future trends, customer behavior, and sales performance. By using historical data and advanced analytics techniques, businesses can forecast potential outcomes and make informed decisions about inventory, marketing, and customer engagement. This proactive approach allows retailers to stay ahead of the competition by anticipating demand and adjusting strategies accordingly.
At its core, predictive analytics uses machine learning algorithms, statistical models, and data mining techniques to analyze vast amounts of historical data. The goal is to uncover patterns, trends, and relationships within the data, which can then be applied to future scenarios. For example, retailers can use retail predictive analytics to predict seasonal spikes in demand, forecast customer purchasing behavior, and optimize pricing strategies.
How Predictive Analytics Helps in Forecasting Demand and Trends
In the ever-evolving retail landscape, accurately forecasting demand is crucial for avoiding stockouts or overstocking, which can lead to missed opportunities or increased costs. With retail predictive analytics, retailers can analyze historical sales data, customer trends, and external factors such as weather patterns or economic conditions to predict future demand. This allows businesses to optimize inventory management, ensuring they have the right products available at the right time.
For instance, by examining past sales data, predictive analytics can identify trends in specific product categories during certain times of the year, such as higher sales of outdoor furniture during the summer or increased demand for holiday decorations in November and December. By recognizing these patterns, retailers can proactively stock their shelves with the right products to meet customer demand.
The Benefits of Proactive vs. Reactive Retail Management
The difference between proactive and reactive retail management is striking. Proactive retail management involves using data and analytics to make decisions before problems arise, while reactive management focuses on addressing issues as they occur. With the help of retail predictive analytics, businesses can take a proactive approach, anticipating challenges before they become costly problems.
For example, predictive analytics can help retailers forecast a dip in demand for certain products or a potential disruption in the supply chain. By identifying these risks early on, businesses can adjust their marketing efforts, reallocate inventory, or find alternative suppliers. In contrast, reactive management only addresses issues after they’ve already impacted sales or operations, resulting in lost revenue, customer dissatisfaction, or unnecessary expenses.
How In Store Analytics Impact Customer Experience
Understanding Customer Behavior Through In Store Analytics
In store analytics focuses on gathering data from customer interactions within a physical store environment. By using technologies such as foot traffic sensors, video analytics, and mobile apps, retailers can gain deep insights into customer behavior, including where customers spend the most time, which products they interact with, and how they navigate the store.
This data provides valuable information about customer preferences, shopping habits, and pain points. For example, in-store analytics can reveal that customers often skip certain sections of the store or tend to linger near high-demand items. Armed with this knowledge, retailers can optimize store layouts, improve product placement, and design more effective in-store promotions that capture customers’ attention and drive sales.
Improving Customer Engagement with Real-Time Data
Real-time data is a powerful tool in enhancing the customer experience. With in-store analytics, retailers can track customer interactions in real-time and respond to changing behaviors instantly. For example, if a customer is lingering near a product but not making a purchase, a store associate can approach them with personalized recommendations or discounts based on their browsing history. Additionally, retailers can use real-time data to adjust pricing, promotions, or product availability based on immediate customer demand.
This level of responsiveness can significantly improve customer satisfaction, leading to higher conversion rates and repeat visits. It also allows businesses to create personalized experiences for customers, which can foster brand loyalty and drive long-term success.
Enhancing In-Store Layouts and Promotions
In-store analytics can also play a crucial role in improving store layouts and designing more effective promotions. By tracking customer movement patterns, retailers can identify high-traffic areas and areas that are underutilized. This insight allows businesses to adjust store layouts to maximize product visibility and encourage customers to explore more products.
For example, if in-store analytics reveals that customers are spending a significant amount of time in the electronics section, retailers can strategically place complementary products, such as phone accessories, nearby to increase cross-selling opportunities. Additionally, in-store promotions can be tailored to target specific customer segments based on their behavior, ensuring that discounts and offers are relevant and impactful.
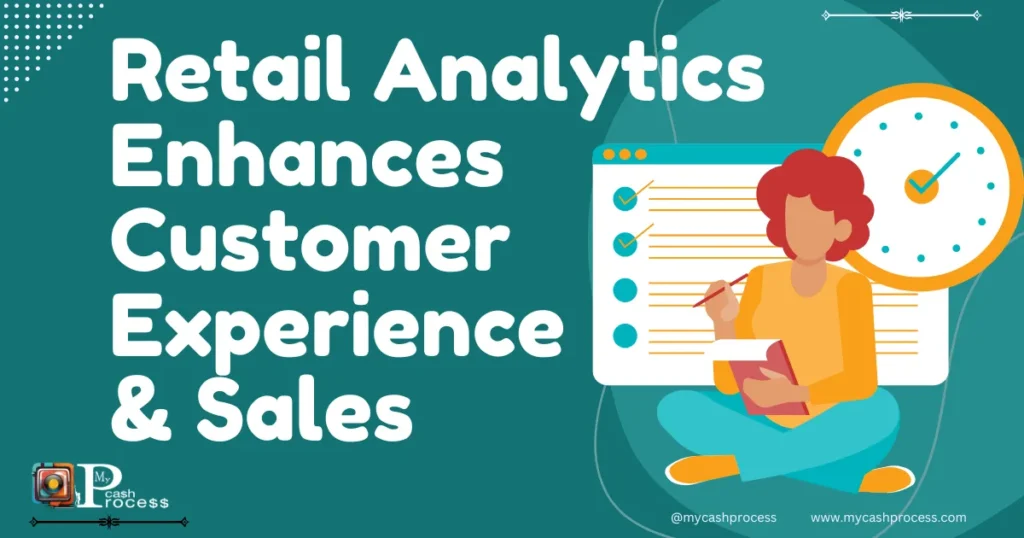
Integrating Data Analytics for Retail: Best Practices
How to Integrate Data Analytics for Seamless Operations
Integrating Data analytics for retail into everyday operations is essential for maximizing efficiency and driving business success. To achieve this, retailers need to adopt a comprehensive approach that unifies various data sources, including sales, customer behavior, inventory, and supply chain data.
The first step in integrating analytics is to establish a centralized data platform that can handle data from multiple sources. This allows retailers to access all relevant information in one place, making it easier to analyze and derive actionable insights. Additionally, businesses should invest in analytics tools and software that can process large volumes of data and generate reports in real time.
Using Multiple Data Sources for a 360-Degree View of Performance
To gain a holistic view of their business, retailers must use data from a variety of sources. This includes sales data, customer behavior data, inventory data, and even external factors such as weather patterns and economic trends. By combining these different data sets, businesses can gain a more complete understanding of their operations and customer needs.
For example, combining sales data with weather data can help retailers forecast demand for certain products. If data shows that warm weather is expected in the coming weeks, businesses can adjust their inventory to include more summer-related products. Similarly, combining customer behavior data with inventory data can help retailers optimize their stock levels, ensuring they have enough products to meet customer demand without overstocking.
The Role of Automation in Data Analysis and Reporting
Automation is key to streamlining data analysis and reporting processes. By automating routine tasks such as data collection, data cleaning, and report generation, retailers can save time and resources while ensuring that their analytics are up-to-date and accurate.
For instance, automated tools can pull in real-time sales data, customer interactions, and inventory levels, allowing businesses to track performance continuously. Additionally, automated reporting can generate custom reports based on specific criteria, such as sales trends or customer demographics, making it easier for retailers to analyze performance and make data-driven decisions.
Maximizing ROI with Retail Analytics
How Retail Analytics Boosts Profitability
Implementing retail analytics has the potential to significantly boost profitability by improving decision-making, optimizing inventory, and enhancing customer experience. By using data to drive decisions, retailers can ensure that they are making the most efficient use of their resources and maximizing revenue opportunities.
For example, predictive analytics can help retailers identify which products are likely to perform well during certain periods, allowing them to stock up on those items in advance and avoid stockouts. Similarly, in-store analytics can help businesses optimize their store layout and product placement, increasing the likelihood of cross-selling and upselling.
Measuring the ROI of Implementing Retail Analytics
To evaluate the success of implementing retail analytics, businesses should track key performance indicators (KPIs) that align with their goals. This may include metrics such as sales growth, customer satisfaction, inventory turnover, and conversion rates.
By comparing these KPIs before and after implementing retail analytics, businesses can determine the ROI of their investment in data-driven tools. For example, if a retailer sees a significant increase in sales or a reduction in inventory costs after adopting predictive analytics, they can confidently conclude that their investment has paid off.
Case Studies of Successful Retail Analytics Implementation
Several retail brands have successfully implemented retail analytics to drive business growth. For example, a global retail chain used predictive analytics to forecast demand for seasonal products, resulting in a 10% increase in sales and a 15% reduction in excess inventory. Another retailer used in-store analytics to optimize its store layout, leading to a 20% increase in customer engagement and a 5% boost in overall sales.
These case studies demonstrate the tangible benefits that retailers can achieve by leveraging data analytics. With the right tools and strategies, businesses can optimize their operations, improve customer satisfaction, and increase profitability.
Challenges in Implementing Retail Analytics
Common Obstacles Faced by Retailers
While retail analytics offers numerous benefits, many retailers face challenges when it comes to implementation. Common obstacles include the complexity of integrating data from multiple sources, the need for specialized expertise to analyze the data, and the potential cost of implementing analytics tools and technologies.
In addition, some retailers struggle with data quality issues, such as incomplete or inaccurate data, which can lead to flawed insights and decision-making. Overcoming these challenges requires a strategic approach to data management and a commitment to continuous improvement.
Solutions for Overcoming Data Analysis Barriers
To overcome the barriers to data analysis, retailers should focus on investing in the right tools and technologies, building a data-driven culture within the organization, and ensuring that their data is clean, accurate, and up-to-date. By working with experienced data analysts or partnering with third-party analytics providers, businesses can improve the quality of their data and ensure that their analytics are accurate and actionable.
Ensuring Data Accuracy and Consistency
Ensuring data accuracy and consistency is critical for successful retail analytics. Inaccurate or inconsistent data can lead to poor decision-making and missed opportunities. Retailers should implement data validation processes to ensure that their data is accurate and complete, and they should establish clear standards for data entry and reporting.
Conclusion
Summary of Key Takeaways from Implementing Retail Analytics
In summary, retail analytics is an invaluable tool for businesses seeking to optimize store performance, improve customer engagement, and drive profitability. By using data-driven insights, retailers can anticipate future trends, optimize inventory management, and create personalized shopping experiences for their customers. The key components of retail analytics, such as retail store analysis report, retail predictive analytics, and in-store analytics, play crucial roles in shaping business strategies and decision-making.
Final Thoughts on Maximizing Retail Success with Retail Analytics
By embracing retail analytics, retailers can unlock new opportunities for growth and innovation. With the right data and tools, businesses can stay ahead of the competition, improve operational efficiency, and maximize ROI. The future of retail is data-driven, and those who leverage retail analytics will be better positioned to thrive in an increasingly competitive market.
Warning
While retail analytics offers many benefits, it is important for businesses to avoid relying solely on data without incorporating human insight. Inaccurate data, flawed analysis, and outdated models can lead to costly mistakes and missed opportunities. Regular updates and adjustments to analytics strategies are essential for staying competitive.
Follow us on Pinterest, Twitter X, Facebook, Instagram, Quora, TikTok, Discord, YouTube, and WhatsApp Channel.
Advice
For businesses looking to integrate retail analytics, it is essential to invest in the right tools, ensure data accuracy, and build a data-driven culture. Additionally, using multiple data sources and automating reporting can streamline the process and enhance decision-making.
FAQs
-
What is the best way to start using retail analytics?
The best way to start using retail analytics is to begin with a comprehensive data strategy. Identify key data sources, invest in the right tools, and focus on improving data quality and consistency.
-
Can predictive analytics really help with inventory management?
Yes, predictive analytics can be incredibly helpful for inventory management. By forecasting future demand based on historical data, retailers can optimize stock levels, reduce excess inventory, and avoid stockouts.
-
Is in-store analytics useful for small businesses?
Yes, in-store analytics can be beneficial for small businesses. With affordable tools and technologies, even small retailers can gain valuable insights into customer behavior, store performance, and sales trends.